Polling errors show why data models need to reach beyond human surveys
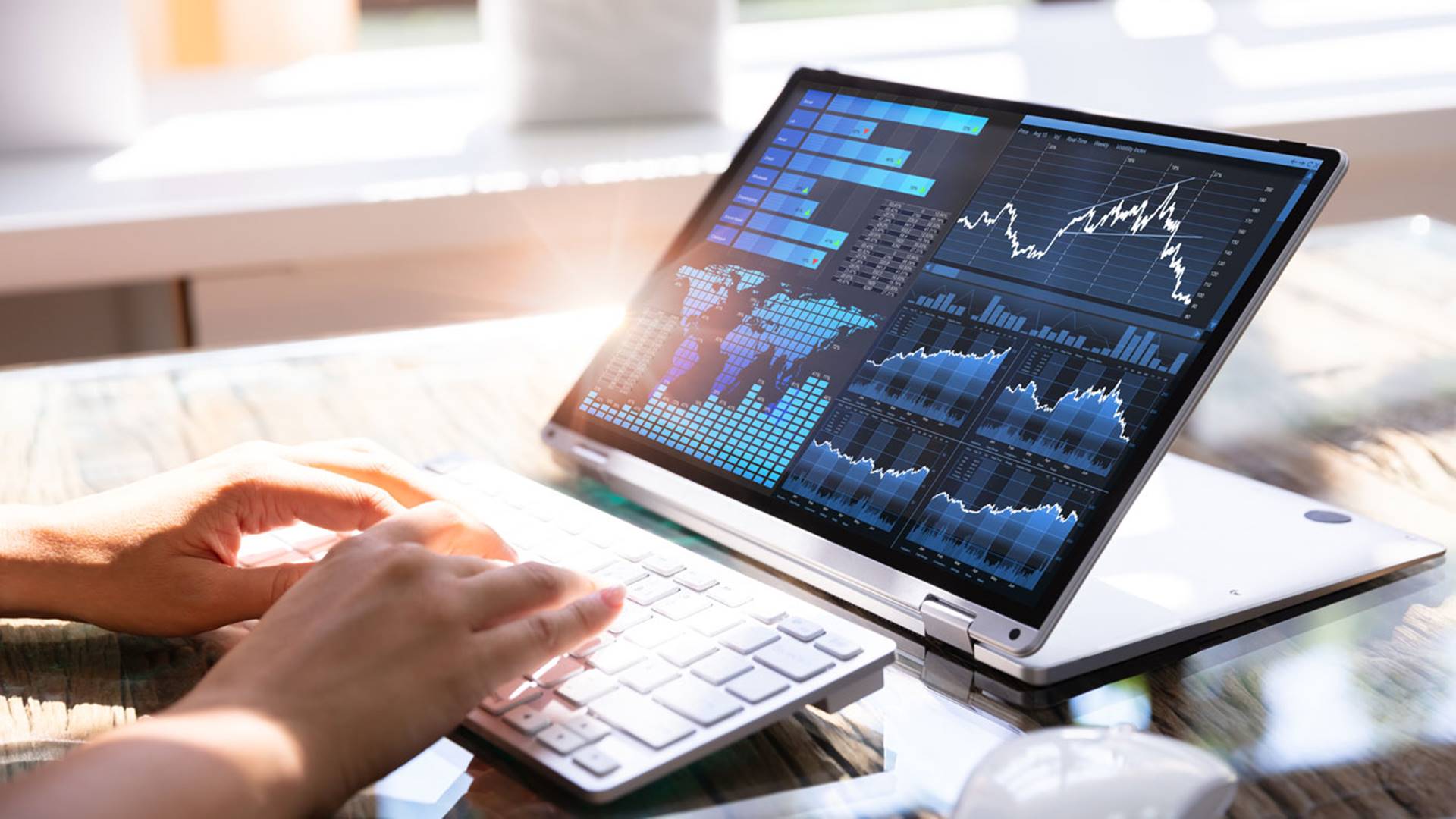
In a tight race, it is very difficult to predict a winner with confidence. The trouble in this election cycle is that most pollsters didn’t even predict that the race would be this tight. Regardless of the actual outcome of the election, one might say that the biggest losers in the 2016 and 2020 election cycles are the pollsters and pundits.
How could they be so far off twice in a row by such large margins? Not just for the presidential election, but for congressional races, too? I, along with many other analytics geeks, attributed sampling errors to the wrong prediction of the last presidential election. For one, when you sample sparsely populated areas, minor misrepresentation of key factors can lead to wrong answers. When voting patterns are divided by rural and suburban areas, for example, such sampling bias amplifies even further.
To avoid the same mistake, I heard that some analysts tried to oversample segments such as “Non-college-graduate White Males” this time. Apparently, that wasn’t good enough, was it? Also, if sample sizes of certain segments are to be manipulated, how did they do it, and by how much? How do analysts make impartial judgments when faced with such challenges? It will be difficult to find just two statisticians who completely agree with each other on the methodology.
Related Posts
Then there are human factors. They say modeling is half-science, half-art, and I used to agree with that statement wholeheartedly. But looking at vastly wrong predictions, I am beginning to think that the art part may be problematic, at least in some cases. Old-fashioned modeling involves heavy human intervention in variable selection and determination of the final algorithm among test models. Statisticians, who are known to be quite opinionated, can argue about seemingly simple matters such as “optimum number of predictors in a model” until cows come home.
In reality, no amount of statistical efforts can safely offset sampling biases, and worse, “wrong” data. An old expression—garbage-in, garbage-out—applies here.
They say modeling is half-science-half-art, and I used to agree with that statement wholeheartedly. But looking at vastly wrong predictions, I am beginning to think that the “art” part may be problematic, at least in some cases.
If a survey respondent did not answer the question honestly, that should be considered as a wrong piece of information. Erroneous data don’t stem only from data collection or processing errors. Some just show up wrong.
The human factor goes beyond model development. When faced with a piece of prediction, how would a decision-maker react to it? Let’s say a weather forecaster predicted that there will be a 60% chance of a shower tomorrow morning. How would a user apply that information? Would he carry an umbrella all day? Would he cancel his golf outing? People use the information the way they see fit, and that has nothing to do with the validity of employed data or modeling methodologies. Would it make a difference if the forecaster was 90% certain about the rain? Maybe. In any case, it is nearly impossible to ask ordinary users to get rid of all emotions when making decisions.
Granted that we cannot expect to eliminate emotional factors on the user side, data scientists must find a more impartial way to build predictive models. They may not have full control over ins and outs of the data flow, but they can transform available data and select predictive variables at will. And they have full control over methodologies for variable selection and model development. In other words, there is room to be creative beyond what they are accustomed to or trained for.
Going back to the example of election result prediction, I am thinking that the days of old-fashioned polling is slowly coming to an end. To put it mildly, predicting the outcome of the election mostly based on phone surveys turned out to be inadequate. In fact, even “phone survey” makes me cringe a little, as “people who picked up the call from an unknown number and answered the survey questions” may be the prime example of sampling bias. Heck, a spam blocker on my phone will stop the phone from even ringing. Maybe it’s a little better than surveying people who visit a particular site, but in this complex world made of a diverse population, prediction primarily based on phone surveys should be treated only as directional guidance at best.
I read a report after the 2016 election that a machine-learning algorithm, which used only empirical data such as “Number of lawn signs in the area”, properly predicted the outcome. Such a variable indicates human behaviors, but it is quite different from “asking someone questions”, which could lead to disingenuous answers.
What people say and what they actually do are often two different things. A while back, my old team was developing a Green Model. Since it was difficult at the time to obtain target data for it, we relied on a syndicated panel survey. At first, we used questions like “Do you support the environmental cause?” as the target definition. Unfortunately, the model fell totally flat. Maybe many respondents figured “Yeah sure, why not?” So, we dug a little deeper and used a series of survey questions regarding “purchases of green products such as solar panels, electric cars, etc.” as targets. Then, the model became much more powerful, and it was later proven to be effective in the market.
There are many ways to find more direct ways to describe human behavior without biases. If a tax collector wants to estimate how many pizzas a pizza shop sold in a year, what would be more accurate and reliable? Just asking the owner (not that I’m accusing all business owners of under-reporting), or obtaining the number of pizza boxes ordered by the owner? If you ask me, I’d always go for the variables with the least amount of human influence. Calling and asking a not-so-randomly-chosen person for whom they are voting? That would be at the bottom of the bias scale.
“Number of lawn signs for each candidate” is a clever example, and there must be many other potential predictors if we look hard enough. To think of a few variables for this case, we can obtain or create variables such as “Number of rally attendees by region”, “Square footage of occupied areas in rallies”, “Number of positive vs negative mentions on social media”, “Number of donations”, “Average donation amount”, “Number of first-time donors vs. repeat donors”, etc., all combined with demographic and geo-demographic data. If such information isn’t available at all, then we must try to be even more inventive. Send in drones if you have to.
In the age of ubiquitous data and machine learning, we can totally expand the set of variables that enter the predictive modeling beyond conventional polling questions (not that I am advocating abandoning surveys completely). Furthermore, models become more powerful when multiple types of variables are used in conjunction with one another.
Modeling efforts for marketing purposes routinely take in hundreds of, if not over a thousand, variables and final algorithms may contain 5-20 different predictors depending on the employed methodologies and preferences of modelers. And nowadays, machine learning can replace many of the manual elements in modeling. Technology isn’t the barrier anymore; lack of imagination and open-mindedness is.
In the age of ubiquitous data and machine learning, we can totally expand the set of variables that enter the predictive modeling beyond conventional polling questions (not that I am advocating abandoning surveys completely).
I emphasized machine learning here, as computers lack emotion. We often think about AI as an automation tool, but when we see predictions that are so widely off the mark, we must be aggressive about eliminating emotional factors in analytics. If not in the usage and story-telling parts, at least in the data manipulation and modeling steps. And not just for prediction of election results every four years, but for everyday analytics, too.
Machines do not come with wishful thinking, and that may be a good thing in data science. Because, ironically, having to deal with wrong predictions is emotionally draining for us humans, especially when the stakes are high.
Adweek / Balkantimes.press
Napomena o autorskim pravima: Dozvoljeno preuzimanje sadržaja isključivo uz navođenje linka prema stranici našeg portala sa koje je sadržaj preuzet. Stavovi izraženi u ovom tekstu autorovi su i ne odražavaju nužno uredničku politiku The Balkantimes Press.
Copyright Notice: It is allowed to download the content only by providing a link to the page of our portal from which the content was downloaded. The views expressed in this text are those of the authors and do not necessarily reflect the editorial policies of The Balkantimes Press.